Abstract
Customer Analytics is this field of analytics, where one dives deep into the consumer data and bring about useful insights on their clients. Customer Analytics find its utmost use in the marketing as well sales departments where the customer data is the key to understand the customer behavior for them chart their marketing as well advertising strategy. Customer Analytics supports business decision making through targeting specific groups based on income groups, age groups, and customer segmentation as understanding customer groups would help the businesses to create more strong strategies for expansion through the use of various predictive models and forecasting methods. It uses parameters like customer spending patterns, customer reviews of different products, customer churn rate to predict customer behavior. The better the understanding of customer habits, the better the predictive models. A good customer analytics platform makes use of all the interdisciplinary departments of a company such as IT, marketing, sales, customer support, and business analysts. A great number of tools such as Enterprise Resource Planning tools, Customer Relationship Management applications and Customer Data Integration tools must be used concurrently and agreed upon to give in a holistic view of the clients. This literature review is aimed at studying at some of the key concepts mentioned above and how the organization uses this information to plan and execute their strategies.Introduction
Data in any form is considered as the new gold in the 21st century. Organizations who primarily focus on the data-driven approaches have the potential to be ahead of their respective game. One of the important and the core objective of any company would be to maintain a solid and strong relationship with its customers, understanding them and providing them what they want. The primary objective of Customer Analytics is to create a distinct and accurate view of customer behavior and patterns, in order to facilitate decision making on how to attract as well as retain customers, along with answering questions like “what is the most suitable product”, “which customer group to target”, “what communication channels must be used”, at what price or point of time, and also what promotional and advertising strategies to be used, paths taken by the clients etc. The organizations are more reliant on analytics due to the ever-increasing sophistication of the customers in their use of technology such as smartphones. On a broader level, customer analytics help the organizations to help find how the customer finds their product, which features they like the most, which features they don’t like and the reasons for leaving whereas, on smaller scale, It helps organizations to identify the customers as individuals by segmenting them on the basis of region, age, income, ethnicity etc. According to (McKinsey & Company, 2018), “Companies that use customer analytics comprehensively report outstripping their competition in terms of profit almost twice as often as companies that do not.” Competitors who make use of tools that gather data from all sources and deliver insights in real-time have a distinct advantage. The use of analytics to know one's customer has been seen of utmost importance by organizations, especially by the customer eccentric companies. It can increase the revenue of organizations through improved customer experience, customer acquisitions, reducing customer churn, maximizing customer lifetime value, and boosting the return on market value. Customer Analytics is in itself is the wide term, incorporating a range of departments such as marketing, sales, analytics, operations, and customer support. Customer Analytics is seen as the one-stop place to all the answers to the customer demands as it incorporates predictive modeling, data visualization, and data management. The stronger the analysis is, the stronger or the better would be the chances of providing good customer experience. Customer Analytics requires that companies support or accelerate a data-driven approach with considerable improvement from the senior management. It is pretty evident that the above analytics need huge amount of data, and with unprecedented amount of data that is being collected through various channels such as social media and mobile applications at an unprecedented speed, it is imperative that organizations leverage Big Data framework to capture, process, analyze and visualize large amounts of real-time data in a limited time frame, hence the term Big Data Analytics consumer analytics. Big Data framework thus enables the usage of unstructured data driven by new communication platforms such social media reviews, mobile application data, images, videos etc along with structured data driven by traditional databases and data warehouses and customer relationship management tools into consideration. .The data then can be subjected to a variety of techniques like statistical modeling, data mining. Optimization methods, data visualizations, and machine learning. Thus Big Data analytics has the potential to change and revolutionize the way organizations use customer data to cater to fast-changing demands of the customers. Customer Analytics can be briefly classified into Customer Journey Mapping and Customer Journey Analytics. Customer Journey mapping would include collecting data on customer journey through visualizations whereas Customer Journey analytics being a data-driven approach would try to connect together every touchpoint of a customer’s journey so as to influence and analyze customer journey and thus prioritize the opportunities that impact the business goals. They both differ from each other in their data-driven approach, in the comprehensiveness, the scale of analytics involved, and usage of real-time data and in presenting actionable insights. The Big Data framework uses a plethora of techniques and tools, explaining all would be out of the scope of this literature review. This literature review would include a methodical review and analysis of the journals written on the same, giving a holistic view of the frameworks, techniques, methods, models, concepts used in customer analytics.Literature Review
Sources of Data
Wedel and Kannan(2016) argue that consumer analytics includes collection, management, and analysis of consumer data using the 3 pillars of analytics that are descriptive, prescriptive and predictive analytics to bring in good return on investments (ROI) through better market insights. Davenport and Harris (2007) also id customer analytics as extensive use of data and models to drive decision making at an individual customer level. This literature review is aimed at reviewing of data-driven approaches or analytical methods are employed in the market while also stressing on the use of Big Data. The word Big Data is always associated with consumer analytics because of the large onslaught of data in terms of velocity, volume, and variety for example sales data, inventory data, social media data and RFID tag (Bradlow, et al., 2017)). The consumer data into five-dimensional space: customer data, products, time, geospatial location and channel. Big Data has the advantage of combining recent data along with the historical data but the historical data of would be too old that it makes the data models and algorithms to perform poorly (Bradlow, et al., 2017). But the positive effects of using Big Data would also include combining information search, recommendation system, dynamic pricing, and customer service into one framework. Data such as like location, customer hobbies, customer likes, dislikes and connections on social media are very much taken into consideration by the E-Commerce companies to provide better customer service through personalized offers and pricing and channels ( Mai L T , Liaw S Y 2017), for example, data such as customer’s region, age, insurance status, sex and customer source have been found beneficial to the insurance industry to optimize profitability (Fang, et al., 2016). Big Data has enabled organizations around the world to switch from the traditional data that is the structured data to the unstructured data for customer analysis. Unstructured data includes textual data from websites, blogs, social media comments and nontextual data of images, videos, and audio have been utilized very efficiently with the use of Big Data framework (Erevelles, et al., 2015).Customer Analytics Methods and Models
The Gartner Ladder is used to explain the methods used in customer analytics. The first step would be to use descriptive analytics as a standard type of analytics to understand and identify consumer data in order to make a good customer relationship management system (Yadav, et al., 2015) . These could include looking into the notable KPIs, such as customer churn, monthly sales growth or net promoter score. Customer behavior patterns are analyzed using predictive analytics in real-time as well as historical data. Diagnostic analytics establishes a relationship between variables and allows for hypothesis testing. Prescriptive analytics makes use of forecasting and risk modeling and optimization to look into the future behavior and this provides insights and recommendations on the same. Predictive analytics and prescriptive analytics makes use of machine learning algorithms like regression, cluster analysis, decision trees, association rules, time series forecasting, linear programming, and optimization techniques. Marketing analysis encounters the use of all four levels of analytic techniques for structured data. Venn diagrams form the very basics of identifying customer segments based on products or channel used to identify relationships and differences. Forecasting time series data identify seasonality and patterns of customer behavior to implement marketing strategies effectively. Association Rules or market-basket analysis as commonly known is widely used in retail industries to identify products that are purchased simultaneously and cross-selling opportunities. Classifying and predicting customer behavior employs decision trees and random forest algorithms. Decision trees are very popular classifying algorithms to predict customer churn and selection of right products for recommending to the customers (Khade, 2016). Predictive models like regression techniques such as linear and logistic regression uses methods such as least square method, method of moments and maximum likelihood estimation whereas sales force allocation, conjoint analysis, product design, and marketing mix application employ the use of optimization models along with use of Bayesian methods, sampling, variable selection methods, Markov Chain Monte Carlo algorithms and parallel computing along for complex models and huge data. Unstructured data such as text, images, and video output are also used extensively to develop metrics associated with customer data. Machine learning techniques such as text mining and sentimental analysis use text mining for customer recommendation and customer behavior analysis for product testing, which is mostly employed by the E-Commerce giants. Unstructured data in customer analytics uses the above methods and metrics to change into structured data due to inefficient application of predictive and prescriptive analytics because of its complexity (Wedel and Kannan 2016). Speaking of Customer Analytics, Recommender systems takes the pole position in terms of its usability and effectiveness in customer retention and customer prediction analysis. Le T M, Liaw S(2017) briefly explains about the use of recommender systems operated by the E-Commerce and Internet giants such as Amazon, Facebook, Netflix and Retails Giants such as Walmart to recommend products based on the current user’s purchasing behavior and also similar purchasing behavior of other users. It uses user-based collaborative filtering and item-based collaborative filtering wherein the user’s based collaborative filtering, it recommends products based on similarity in user ranking and item-based collaborative is based on the description of the item and user’s ranking on the same. (Alyari & Navimipour, 2018) explains the concepts and methods used in recommender systems such as Content-based recommender system, collaborative recommender system, Cold-start recommender system, demographic recommender system, hybrid recommender system, knowledge-based recommender system. Content-based recommender system stresses on recommending products based on the similarity of user’s choices with the target user. Content-based recommender system uses mapping of each item to the user‘s historical data of preferences to rate the user. It then matches the products with the user’s profiles. Cold start technique recommends products to the new users based on the popular product choices among the customer base. Metrics such as age, location, gender, user activity of similar pages are used to recommend products Recommender systems uses different machine learning and clustering algorithms such as K-Means algorithm, association rules, and other clustering techniques. Association rules recommend products based on user’s purchasing behavior and what they put in the cart. Text analytics is been used at the forefront of customer analytics as discussed above, predominantly by the E-Commerce giants such as Facebook, Amazon, Netflix for predicting customer behavior. The market for text analytics will reach $5.93 billion by 2020, with 80% of enterprise data being unstructured at the same time identifying four distinctive features of modern text analytics (Muller O et al 2016). Data-driven approach of modern Text analytics has given it self-adaptability i.e the ability to learn with more data. Statistical machine learning algorithms are being used to discover patterns such that it can take ungrammatical text from emails, post, user posts and comments on social media. Real-time analysis of textual data has also been employed by organizations to analyze the customer sentiment in real time with the APIs provided by the internet giants, where one could access gigabytes of data at one go such as that of Twitter and Facebook. Modern text analytics is said to provide probabilistic inferences rather than giving out deterministic inferences, which provides many answers to a single query. Visual analytics such as word cloud, word frequency histograms have been used extensively along with text analytics that communicates the results in a spontaneous way, readable and understandable by non-specialists. The four distinctive features have been shown below :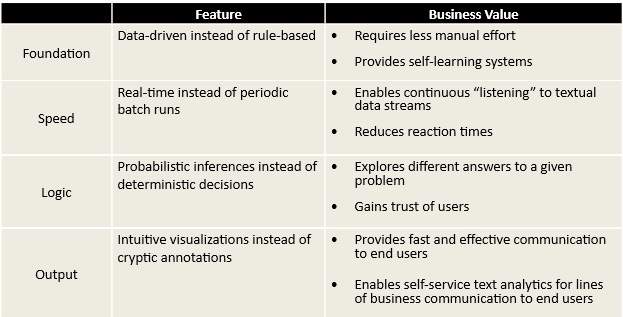
Conclusion
This literature review was aimed at providing an intuitive use of customer analytics in an organization, its applications and the different models used. The first part showed how and what kind of data is used along with the definition of Big Data in terms of customer data. The second part discusses the different models and machine learning algorithms used in customer analytics. The third part was focused on the applications of customer analytics on the organizations. There is no denying the fact that there are positive as well negative effects on the effective implementation of customer analytics. Huge data also cater to the problem of compensating on data quality with data size. Huge data sets come with a lot of historical data that are not relevant to the present scenario in the market, which may lead to an incorrect prediction of the model. Owing to the complexity of the machine learning algorithms, choosing inappropriate algorithms also leads to biasing and incorrect predictions or analysis. Thus in the present digital age, with the huge inflow of data, it is imperative that correct use of quality data along with proper models would give the competitive edge for the organizations in this customer eccentric environment.References
McKinsey & Company. (2018). Why customer analytics matter. [online] Available at: https://www.mckinsey.com/business-functions/marketing-and-sales/our-insights/why-customer-analytics-matter [Accessed 14 Nov. 2018].
Cite This Work
To export a reference to this article please select a referencing style below: